Data-based Analysis of Dwelling Plans in London
Year 2020-2021
Team Seyithan Ozer (Lead Researcher), Wojciech Mazan (Researcher), Sam Jacoby (Research Supervisor)
Project Partners XCYDE, Archilyse, Archilogic
Funder Prosit Philosophiae Foundation
This pilot study digitises a sample of dwelling plans in London aided by machine learning to create semantic models for analysis. It evaluates the quality of available floor plan data, sampling methodology, and transferability of the approach. It further compares data-based analysis to conventional case and typological studies of housing.
The study examines differences and variations of housing design using a statistical and dimensional approach. It uses morphological criteria to test the limitations of common typological and qualitative methods of analysis that are often used in practice to determine design decisions.
Morphological criteria are more commonly used in regulatory instruments to generalise common formal aspects, while in contrast typological classifications or property types are socio-culturally defined. A morphological analysis provides a more direct evaluation of housing design criteria and is essential to analysing functional requirements at the dwelling scale.
A great deal of attention has been historically paid to the dimensioning of dwellings to safeguard minimum space standards and assess the qualitative aims of creating ‘better’ or ‘well-designed’ housing, whose meanings have changed over time. Relying on standard plans that are repeated or generic or typical plans, indicating preferred or common design solutions that can be followed but also interpreted to meet design standards, this interrelation between standards and standardised plans has reinforced typical dwelling unit layouts.
Based on the analysis of 2,283 dwelling plans sampled from different London boroughs, the study explores how a large dataset provides new insights into design regulation, processes, and decisions. The study asks if this challenges or supports commonly held qualitative design assumptions, in particular, if this changes the relationships between quantitative and qualitative criteria underpinning housing evaluations, housing policy, and common typological classifications?
Year 2020-2021
Team Seyithan Ozer (Lead Researcher), Wojciech Mazan (Researcher), Sam Jacoby (Research Supervisor)
Project Partners XCYDE, Archilyse, Archilogic
Funder Prosit Philosophiae Foundation
Findings
-
Compared to London Design Guide (2010), 61% of dwellings failed the recommended minimum GIAs. Overall, 22% of dwellings were up to 10%, 31% of dwellings were between 10% and 25%, and 8% of dwellings were more than 25% smaller than the prescribed gross internal floor areas.
-
63% of studios were below the required 37m² standard, with 22% being up to 10% (33.3-37m²), 27% between 10 and 25% (27.8-33.3m²), and a significant 14%, more than 25% below (<27.8m²). In addition, 10% of one-bedroom dwellings were also below 37m².
- This study further found that 58% of dwellings fail the desirable criteria – bedroom and living area sizes of London Design Guide (2010). Most dwellings failing dwelling size standards also fail desired room sizes.
- Compared to historical standards, most of which were for two-storey, three-bedroom family dwellings and public sector housing, it was found that many dwellings, at the time they were built, were smaller than the space standards.
- However, a general correspondence between dwelling size and space standards was, evident for housing built by both the public and private sectors, showing the relationships between space standards and the prevalent dwelling size norms.
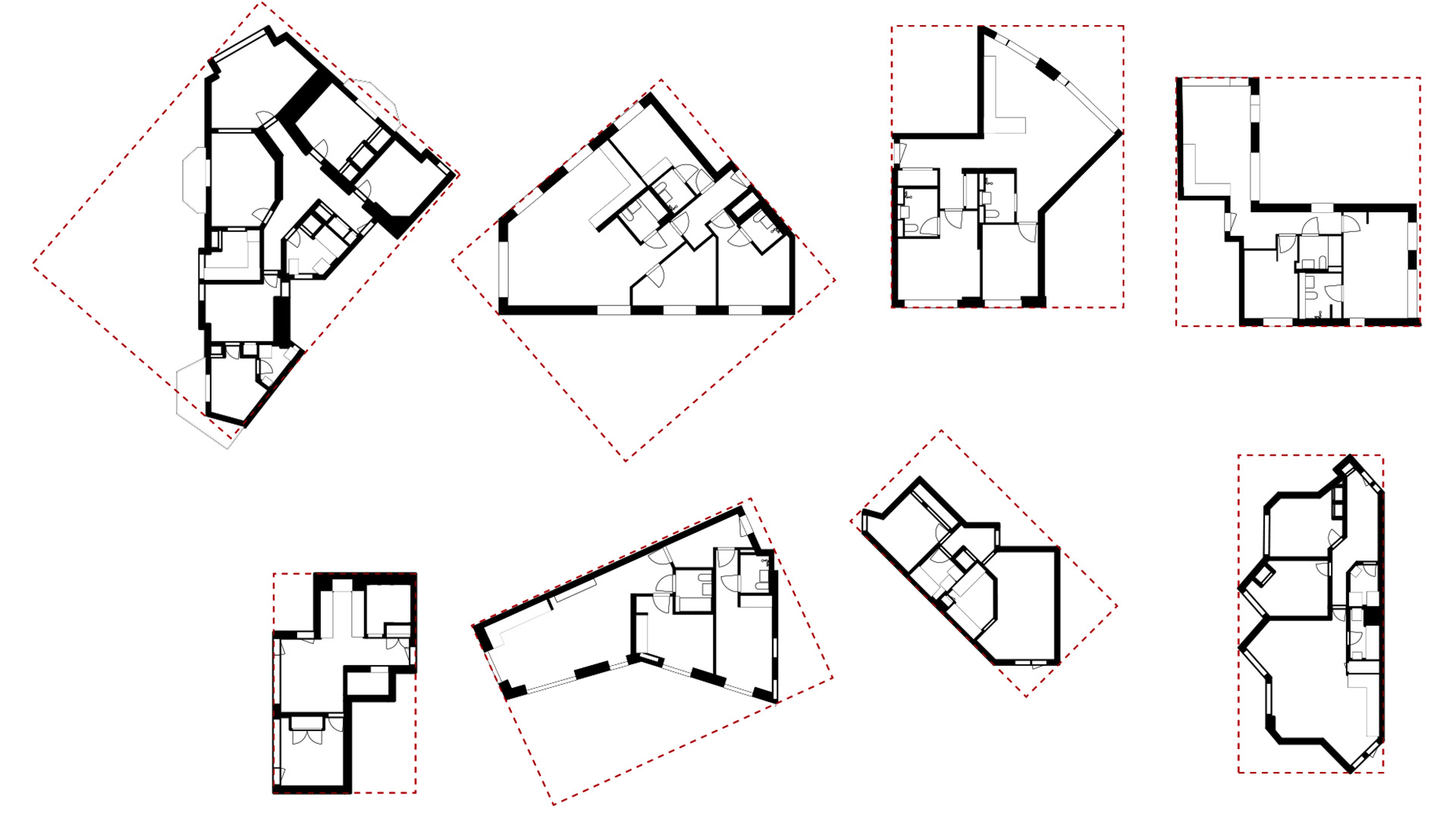
Dwelling plans with low compactness ratios
Outputs
Özer, S. & Jacoby, S. (2022). Dwelling size and usability in London: A study of floor plan data using machine learning. Building Research & Information.